In this blog article, I'll explore the differences between LLMs and traditional chatbots, the advantages of LLM-based systems such as conversational chatbots, and the important role that human customer service representatives still play in customer service today.
As customer service becomes increasingly digital, the use of chatbots has become a popular solution for handling routine inquiries and providing quick answers to customers.
While traditional chatbots are limited by their rule-based systems or decision trees, a new type of AI model known as the Large Language Model (LLM) has emerged as a more versatile and advanced alternative.
What are LLMs and how do they differ from traditional chatbots?
Traditional chatbots are typically built using rule-based systems or decision trees, which means that they can only respond to specific prompts or questions with pre-programmed responses.
A Large Language Model (LLM) is a type of Artificial Intelligence (AI) model that is trained on large amounts of text data. This training allows the LLM to generate human-like text, which makes it more versatile and able to understand and respond to a wide range of customer inquiries in a more personalised way.
This is the key practical difference between a traditional chatbot, where a human has to have written the responses for the bot, and an LLM-based bot that can generate those replies itself based on its training data.
LLM chatbots in customer service
In terms of customer service, LLMs have several key advantages over traditional chatbots:
LLMs can understand and respond to customer inquiries in a more natural and human-like way, which can lead to better customer satisfaction.
LLMs can handle more complex or open-ended questions, which traditional chatbots may not be able to answer.
LLMs can be trained on a company's specific customer service data, allowing them to understand and respond to customer queries in a more personalised way.

In contrast to traditional chatbots, this is also where the dangers lie. Traditional chatbots are deterministic in their responses, meaning that all the responses are crafted based on a certain input and that input will always generate the same response.
In contrast to traditional chatbots, this is also where the dangers lie.
LLMs, however, are probabilistic. They predict the next word based on the previous words it has received and does not follow any predetermined question-answer patterns. As has been seen with ChatGPT, there is no guarantee that the answer an LLM chatbot gives is factual.
Conversational chatbots
It's clear that moving from a deterministic to a probabilistic conversational chatbot is going to be a drastic change. It’s going to require not only technical changes but changes to the way human support agents work as well.
A conversational chatbot will inevitably be better than the current crop of rule-based chatbots in handling simple informative queries. Many customer queries are focused on simply finding the correct answer to their problem.
Some of the common topics for customer support are:
Account information: Customers ask about their account status, billing information, or how to update their account details.
Product or service information: Customers ask about features, specifications, or compatibility of products or services.
Technical support: Customers ask for help with technical issues, such as how to set up or troubleshoot a product.
Order tracking: Customers ask about the status of an order, shipping details, or how to cancel or modify an order.
Returns and exchanges: Customers ask about return policies, how to initiate a return or exchange, or the status of a return.
Privacy and security: Customers ask about privacy policies, security measures, or how to report a security issue.
General inquiries: Customers ask about company policies, hours of operation, or other general information.
A properly configured conversational chatbot can craft an answer which will exactly match the customer's question and lead to better customer satisfaction in all of the above categories.

Many customer queries are focused on simply finding the correct answer to their problem.
For example, technical problems often require a lot of background information and the ability to merge information from many different sources. Instead of relying on existing answers and FAQ pages to point to the customer, a conversational chatbot can craft the answer from multiple sources. What's more, it can deal with more complex and open-ended questions where traditional chatbots would fall short.
Where the conversational chatbot will inevitably have difficulties are cases where an absolute answer or human judgment is required.
LLM-based models have a tendency to “hallucinate”, i.e. come up with answers which are not correct but sound authoritative. This is going to be a problem in high-stakes situations like giving detailed technical instructions to the customer.
Human judgment, on the other hand, is called for in all cases where answering the customer query will result in consequential actions, like choosing how much credit to give to the customer. At least for now, AI solutions do not exhibit creative problem-solving capabilities or show initiative in solving customer problems beyond the expected.
Where the conversational chatbot will inevitably have difficulties are cases where an absolute answer or human judgment is required.
For these types of questions, there will still be a need for humans to answer customer support questions.
The role of humans in customer service
First-line customer support is a critical component in any customer support operation, as it sets the tone for the customer's overall experience with the company and can have a significant impact on customer satisfaction and loyalty.
Conversational chatbots are a great addition to this first-line support where they can provide answers for a wide range of issues in a more natural and human-like way, which can lead to better customer satisfaction.
However, there will still be a need for human-provided support in some situations, like these:
Emotional support: Even conversational chatbots may not be equipped to handle emotionally charged or sensitive issues, such as complaints or concerns about a product or service, and may not be able to provide the empathy and understanding that customers need in these situations.
Legal or regulatory inquiries: The probabilistic nature of conversational chatbots means that they may not be able to handle complex legal or regulatory questions in a safe way. These types of questions are high-stakes as wrong answers can lead to litigation or fines, so using a probabilistic approach is risky.
Unique situations: Unique or unexpected situations that fall outside of the material they were trained on and may require the flexibility and creativity of a human customer support representative.
All the situations described above are something a chatbot, even a conversational one, will have trouble dealing with. In addition, there are situations where jurisdictions like GDPR prohibit purely automatic decision-making, so there is a need for a human to be part of the process.
AI solutions powering agents to provide better customer service
This doesn't mean that there isn't a role for advanced AI solutions like conversational chat in the second-line support.
In fact, one of the most exciting use cases for AI solutions is to help customer service agents to answer larger pools of questions quicker and better than they otherwise could. If a conversational chatbot can help customers to find and digest information, there is no reason it can't do the same for the service agent.
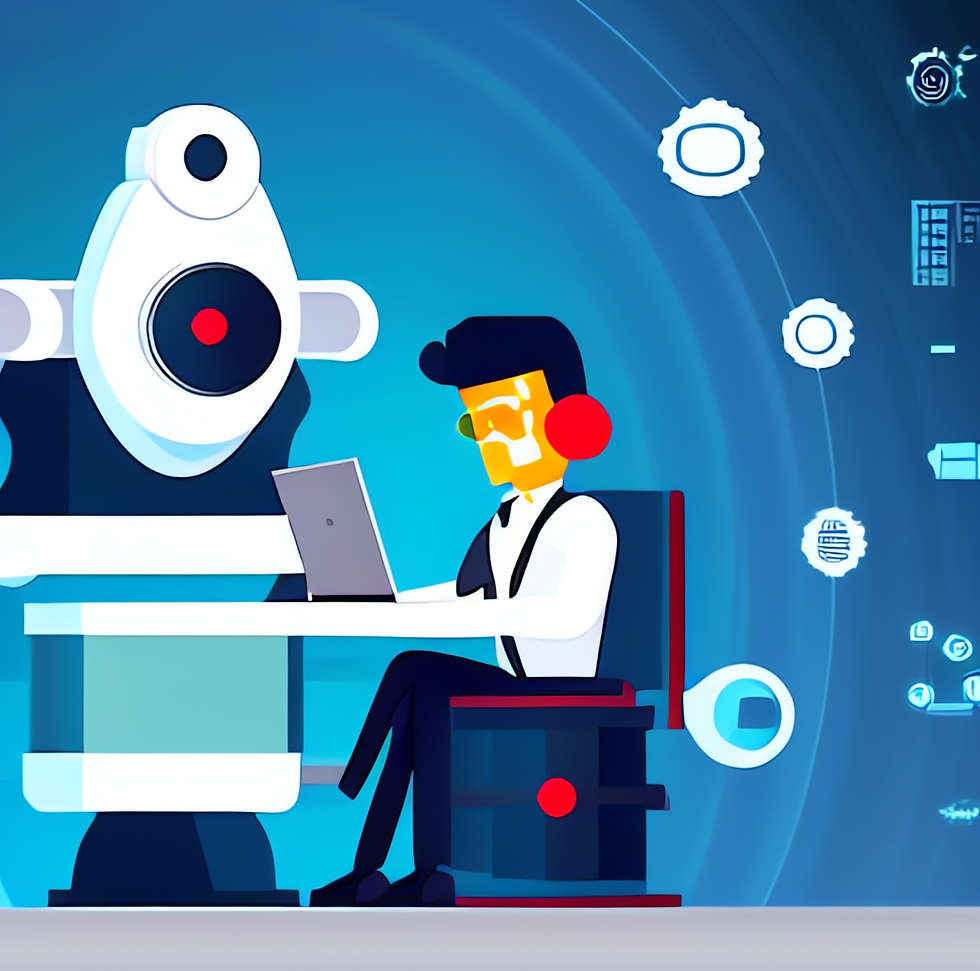
Some of the ways an advanced LLM-based AI can help support agents:
Real-time translation: real-time translation of customer inquiries and responses allow customer support agents to communicate effectively with customers who speak different languages.
Suggested responses: AI can provide suggested responses to customer inquiries based on the customer's request and the information available in the company's knowledge base, allowing customer support agents to respond quickly and accurately.
Automated data retrieval: automatically retrieving relevant information from the customer's account or other systems using AI will reduce the time it takes for customer support agents to gather the information they need to resolve customer inquiries.
Contextual understanding: LLM-based AI systems can provide customer support agents with a deeper understanding of the customer's issue by analysing the context of the inquiry, allowing them to respond more effectively and efficiently.
One of the most exciting use cases for AI solutions is to help customer service agents to answer larger pools of questions quicker and better than they otherwise could.
By leveraging the capabilities of an LLM-based AI, human customer support agents can provide faster and more effective support to customers, improving the overall customer experience and reducing the workload on the support team.
Conclusions
Large Language Model (LLM) based AI systems are revolutionising the customer service industry, providing a more natural and human-like way to respond to customer inquiries.
However, it is important to understand that these systems are still probabilistic in nature and may not always provide accurate answers. In certain cases, there will still be a need for human support to handle emotionally charged or sensitive issues, complex legal inquiries, and unique or unexpected situations.
Companies that effectively leverage AI technology in their customer service operations are poised to provide a better customer experience, leading to increased customer satisfaction and loyalty.
While conversational chatbots can provide efficient and effective support for many routine customer inquiries, human customer support representatives will still play an important role in ensuring that customers receive the support they need.
What's often forgotten by company executives is the capability of AI to transform not only first-level customer support but also to enhance the capabilities of their existing workforce.

In the end, the rise of LLM-based AI systems is a positive development for the customer service industry, providing customers with more effective and efficient support, freeing up human representatives to focus on the most complex and sensitive customer inquiries while providing them with better tools to handle those demanding cases.
Companies that effectively leverage AI technology in their customer service operations are poised to provide a better customer experience, leading to increased customer satisfaction and loyalty.
See how Fluentic uses AI to help customer support agents be more productive.
Author: Anssi Ruokonen, CPO at Fluentic and AI Doctoral Researcher at the University of Helsinki.
anssi.ruokonen@fluentic.com
An LLM-based ChatGPT was used to augment the author's capabilities when writing this article. All images in this article have been generated with AI.
Commenti